Welcome to MicroSci Publisher
Environmental microbial diversity and ecosyste...
Microbiome and Chronic Diseases: Association, ...
Relationship between HIV Mutation and Host Ant...
Application of Artificial Intelligence in Earl...
Interaction between Pathogenic Mechanism of S...
Exploring the Evolutionary History of Differe...
The Rise of Bt Genetically Modified Crops and ...
Tradition and Modernity: Evolution of China Li...
- Queensland researchers have discovered that a mutat...(23 Apr.2024)
- Dr. Mustafa Akbulut, professor of chemical engineer...(23 Apr.2024)
- Researchers report February 15 in the journal Cell ...(23 Apr.2024)
- A study shows that the making of a delicious cup of...(23 Apr.2024)
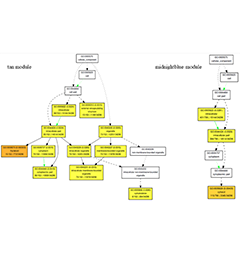
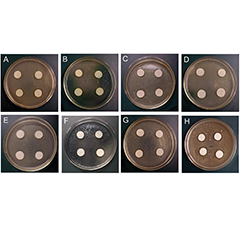
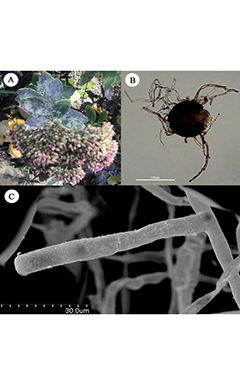
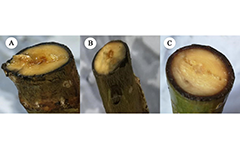